Understanding the Mechanics of AI Image Generators
Introduction to AI Image Generators
Artificial intelligence has revolutionized numerous fields, and the realm of image creation is no exception. AI image generators are powerful tools that create images from textual descriptions or other input data. These generators utilize complex algorithms to produce high-quality images, making them increasingly popular in various industries. The search query “AI image generators” and related keywords like neural networks, deep learning, GANs, machine learning, and image synthesis are central to understanding how these AI systems work.
AI image generators primarily rely on neural networks and deep learning technologies. Neural networks are computational models inspired by the human brain that can recognize patterns and learn from data. Deep learning, a subset of machine learning, involves large neural networks with many layers that can preprocess and analyze vast amounts of information. Together, these technologies facilitate the process of generating images by AI systems.
The evolution of AI image generators is marked by the development of Generative Adversarial Networks (GANs). GANs consist of two neural networks, the generator and the discriminator, which work together to produce realistic images. The generator creates images, while the discriminator evaluates them against real-world data. This continuous training loop results in improved image quality over time.
Understanding the mechanics behind AI image generators involves delving into the intricacies of these technologies and their applications. From creating artwork to aiding in scientific research, AI image generators are proving to be valuable tools with transformative potential.
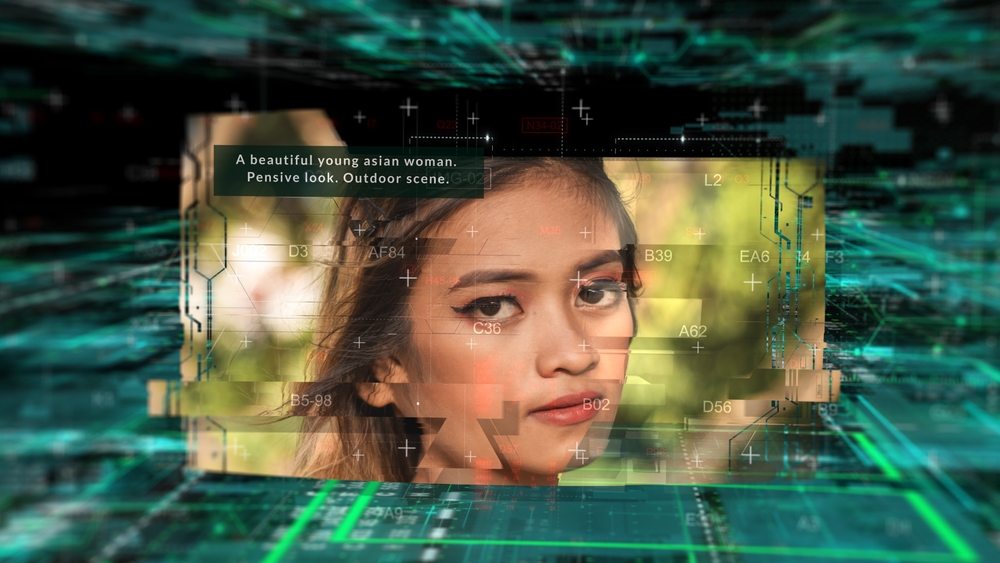
How AI Image Generators Work
AI image generators function by translating input data into visual images. This process begins with a neural network that processes the input, whether it be textual prompts, sketches, or other data. The network then applies learned patterns and data to create a coherent image. The search query “AI image generators” aligns with this transformation process, which is driven by machine learning and deep learning technologies.
Generative Adversarial Networks (GANs) are a cornerstone of AI image generation. These networks use a two-part system: the generator and the discriminator. The generator creates images based on the input, while the discriminator assesses the images’ authenticity compared to real images. Through iterative training, the generator improves its output, leading to more realistic and accurate image synthesis.
Machine learning is crucial in shaping the capabilities of AI image generators. By training on extensive datasets, algorithms learn the intricacies of visual elements and styles, allowing them to recreate and innovate in image production. This ability to learn and adapt is what makes AI image generators powerful tools in various domains, from creative industries to medicine.
Deep learning frameworks, such as TensorFlow and PyTorch, provide the infrastructure needed to build and train AI models that generate images. These frameworks support complex calculations and data processing, crucial for the high demands of AI-driven image synthesis. Understanding these components helps demystify the processes that enable AI to produce lifelike images.
Applications of AI Image Generators
AI image generators have diverse applications across different sectors. In the entertainment industry, they are used to create digital artwork, animations, and special effects for movies and video games. These generators can produce images that would be arduous and time-consuming to create manually, making them invaluable in creative production.
The search query “AI image generators” also finds relevance in marketing and advertising. Businesses use AI-generated images for branding, product design, and promotional materials. The adaptability of these tools allows for customization and rapid production, which is essential in fast-paced market environments.
In healthcare, AI image generators play a role in diagnostic imaging and medical research. By generating synthetic images for training purposes, these tools enhance machine learning models’ performance in detecting and diagnosing diseases. This application underscores the importance of machine learning and deep learning in advancing medical technology.
Further, architects and designers use AI image generators for conceptualizing and visualizing projects. These tools allow professionals to explore new concepts and present them in a visually compelling manner. The ability to generate realistic renderings helps in communicating ideas effectively and streamlining the design process.
Challenges and Ethical Considerations
While AI image generators offer remarkable benefits, they also pose significant challenges and ethical questions. One major concern is the potential for misuse in creating deepfakes or misleading imagery. These applications raise issues of trust and authenticity, as AI can produce images that are indistinguishable from reality.
There are also concerns regarding intellectual property and ownership of AI-generated images. As these systems can create new images based on existing data, questions arise about who holds the rights to the generated content. This area of AI law is still evolving and presents challenges as technology advances.
Another ethical consideration is the impact of AI on employment in creative industries. While AI image generators enhance productivity, they also threaten jobs traditionally held by human artists and designers. Balancing AI integration with human creativity is a topic of ongoing discussion in the AI community.
Finally, the carbon footprint of training and operating AI models is significant. As AI image generators require substantial computational power, they contribute to increased energy consumption. Developing more efficient algorithms and using renewable energy sources are potential solutions to mitigate this impact.
Conclusion
AI image generators represent a significant leap forward in the field of artificial intelligence, offering diverse applications and transformative potential. By harnessing the power of neural networks, deep learning, GANs, machine learning, and image synthesis, these tools redefine how images are created and utilized across industries. However, as with any technological advancement, they come with challenges and ethical considerations that require careful navigation. Balancing innovation with responsibility will be key to realizing the full potential of AI image generators while addressing their broader impacts.